Patient-Generated Data in Precision Medicine
Patient-generated data (PGD) refers to health-related information created, recorded, or gathered by patients themselves, rather than by healthcare providers. This data encompasses a wide range of information, from daily health logs and wearable device outputs to patient-reported outcomes and personal health records. The rise of digital health technologies has significantly increased the volume and variety of patient-generated data, making it a valuable resource for healthcare providers and researchers.
The importance of patient-generated data lies in its ability to provide a comprehensive and continuous picture of an individual's health. Unlike traditional clinical data, which is often collected during sporadic healthcare visits, PGD offers real-time insights into a patient's daily life and behaviors. This continuous stream of data can enhance the understanding of a patient's health status, improve the accuracy of diagnoses, and support more personalized and effective treatment plans. In the context of precision medicine, which aims to tailor medical care to the individual characteristics of each patient, PGD is particularly valuable.
Role in Precision Medicine
Precision medicine seeks to customize healthcare by considering individual variability in genes, environment, and lifestyle. Patient-generated data plays a crucial role in this approach by providing detailed and personalized information that complements traditional clinical data. By integrating PGD with genetic, biomarker, and other clinical data, healthcare providers can develop a more holistic view of a patient's health. This comprehensive perspective allows for more accurate predictions of disease risk, tailored interventions, and better management of chronic conditions.
Additionally, patient-generated data can help identify patterns and trends that might be missed during routine clinical visits. For example, data from wearable devices can reveal fluctuations in a patient's heart rate, activity levels, or sleep patterns, providing early indicators of potential health issues. Patient-reported outcomes can offer insights into the effectiveness of treatments from the patient's perspective, highlighting areas for improvement and personalization. Overall, PGD enhances the precision medicine approach by adding depth and detail to the understanding of individual health.
Types of Patient-Generated Data
Health Records, Wearable Device Data, Patient-Reported Outcomes
Health Records: These include personal health records maintained by patients, which can encompass medical histories, medication logs, and vaccination records. Patients often use health apps and online portals to track their health information, which can be shared with healthcare providers to ensure comprehensive and coordinated care.
Wearable Device Data: Wearable devices such as smartwatches, fitness trackers, and continuous glucose monitors generate a wealth of health-related data. This includes metrics like physical activity levels, heart rate, sleep patterns, and blood glucose levels. Wearable device data provides continuous and real-time monitoring, offering valuable insights into a patient's daily health behaviors and physiological responses.
Patient-Reported Outcomes (PROs): PROs are reports directly from patients about their health conditions and treatment experiences. These can include symptoms, quality of life measures, pain levels, and satisfaction with care. PROs are often collected through surveys, health diaries, and mobile apps, providing subjective insights that are crucial for understanding the patient's perspective and tailoring care accordingly.
Examples and Sources of Data
Examples of Patient-Generated Data:
- A patient using a mobile app to log daily blood pressure readings.
- A fitness tracker recording steps taken, calories burned, and sleep quality.
- A digital diary where a patient records symptoms and side effects of a new medication.
- Patient feedback on treatment effectiveness and quality of life collected via online surveys.
Sources of Data:
- Wearable Devices: Smartwatches, fitness trackers, continuous glucose monitors, heart rate monitors.
- Mobile Health Apps: Apps for tracking diet, exercise, medication adherence, symptom logging.
- Online Portals and Health Records: Personal health records maintained through healthcare provider portals or standalone health apps.
- Surveys and Diaries: Digital or paper-based surveys, health diaries, and questionnaires.
Benefits for Precision Medicine
Enhancing Personalized Care and Treatment Plans
Patient-generated data significantly enhances personalized care by providing detailed and real-time information about a patient's health. This data allows healthcare providers to tailor treatment plans to the individual's specific needs and circumstances. For example, continuous glucose monitoring data can help diabetics adjust their insulin doses more precisely, leading to better glycemic control and reduced risk of complications. Similarly, wearable devices that track physical activity and sleep can inform lifestyle interventions for patients with cardiovascular conditions or sleep disorders.
By integrating PGD with genetic and clinical data, providers can create comprehensive and dynamic treatment plans that evolve with the patient's health status. This personalized approach not only improves the effectiveness of treatments but also reduces the likelihood of adverse effects. For instance, pharmacogenomic data combined with patient-reported outcomes can guide medication choices and dosing, minimizing side effects and enhancing therapeutic efficacy.
Improving Patient Engagement and Outcomes
Patient-generated data fosters greater patient engagement by encouraging individuals to take an active role in managing their health. When patients track their own health metrics and see the impact of their behaviors and treatments, they are more likely to adhere to prescribed regimens and make healthier lifestyle choices. This active participation is crucial for managing chronic conditions and achieving long-term health goals.
Moreover, PGD provides a platform for continuous feedback between patients and healthcare providers. Patients can report their experiences and outcomes in real-time, allowing providers to make timely adjustments to treatment plans. This ongoing communication and collaboration enhance the patient-provider relationship and lead to better health outcomes. Studies have shown that patients who are actively engaged in their care tend to have better adherence to treatments, improved health behaviors, and higher satisfaction with their care.
Data Collection Methods
Technologies and Tools for Data Collection
The collection of patient-generated data is facilitated by a variety of technologies and tools designed to capture and transmit health information efficiently and accurately. These include:
- Wearable Devices: Devices like smartwatches, fitness trackers, and medical sensors continuously monitor physiological parameters such as heart rate, activity levels, sleep patterns, and glucose levels. These devices often sync with mobile apps or online platforms, allowing patients and providers to access and analyze the data.
- Mobile Health Apps: Health apps enable patients to log their health metrics, track medication adherence, record symptoms, and report outcomes. Apps can also integrate with wearable devices to provide a comprehensive view of the patient's health.
- Remote Monitoring Systems: These systems use connected devices to monitor patients' health conditions remotely. For example, blood pressure monitors and weight scales can transmit data to healthcare providers, facilitating ongoing management of chronic diseases.
- Digital Surveys and Diaries: Online surveys and health diaries allow patients to report their symptoms, treatment experiences, and quality of life. These tools can be customized to collect specific data relevant to the patient's condition and treatment plan.
Ensuring Accuracy and Reliability of Data
To ensure the accuracy and reliability of patient-generated data, it is essential to use validated tools and implement standardized protocols for data collection. Key strategies include:
- Device Validation: Using wearable devices and sensors that have been validated for accuracy and reliability through clinical studies. This ensures that the data collected is trustworthy and comparable to clinical measurements.
- Standardized Data Entry: Providing clear instructions and standardized formats for patients to log their health information. This reduces variability and errors in data entry, improving the consistency and usability of the data.
- Regular Calibration and Maintenance: Ensuring that devices are regularly calibrated and maintained to prevent drift and inaccuracies in measurements. This is particularly important for devices like glucose monitors and blood pressure cuffs.
- Data Verification: Implementing systems for verifying and cross-checking patient-generated data against clinical data. For example, periodic in-clinic measurements can be compared to remote monitoring data to ensure consistency and accuracy.
Data Integration and Analysis
Combining Patient-Generated Data with Clinical Data
Integrating patient-generated data with clinical data is essential for creating a comprehensive and accurate picture of a patient's health. This integration involves combining data from various sources, such as electronic health records (EHRs), wearable devices, and patient-reported outcomes, to provide a holistic view of the patient's health status.
Steps for Data Integration:
- Data Aggregation: Collecting data from multiple sources, including EHRs, wearable devices, and mobile health apps, and aggregating it into a central repository.
- Data Standardization: Standardizing the format and structure of the data to ensure compatibility and interoperability between different systems. This involves using common data standards and protocols, such as HL7 and FHIR.
- Data Harmonization: Reconciling differences and inconsistencies between data sources to create a unified and coherent dataset. This may involve data cleaning, normalization, and transformation processes.
- Data Integration: Integrating the harmonized data into the patient's EHR, allowing healthcare providers to access and analyze the data in conjunction with clinical information.
Analytical Tools and Techniques
Advanced analytical tools and techniques are used to process and analyze patient-generated data, transforming raw data into actionable insights. These tools include:
- Data Analytics Platforms: Comprehensive analytics platforms that integrate data from multiple sources and provide tools for data visualization, analysis, and reporting. These platforms can identify trends, patterns, and correlations in the data, supporting informed decision-making.
- Machine Learning Algorithms: Machine learning algorithms can analyze large datasets to identify patterns and predict health outcomes. For example, predictive models can use patient-generated data to forecast disease progression, identify risk factors, and recommend personalized interventions.
- Natural Language Processing (NLP): NLP techniques can analyze unstructured data, such as patient-reported outcomes and free-text notes, to extract meaningful information. This allows for the inclusion of qualitative data in the analysis, providing a more comprehensive understanding of the patient's health.
- Data Visualization Tools: Data visualization tools create intuitive and interactive visual representations of the data, making it easier for healthcare providers to interpret and act on the insights. Dashboards and charts can highlight key metrics and trends, facilitating quick and informed decision-making.
Privacy and Ethical Considerations
Protecting Patient Privacy and Data Security
Protecting the privacy and security of patient-generated data is paramount. Given the sensitive nature of health information, robust measures must be implemented to ensure that data is kept confidential and secure. Key strategies include:
- Data Encryption: Encrypting data both in transit and at rest to prevent unauthorized access and breaches. Encryption ensures that even if data is intercepted, it cannot be read without the appropriate decryption keys.
- Access Controls: Implementing strict access controls to ensure that only authorized individuals can access patient-generated data. This includes role-based access controls, multi-factor authentication, and regular audits of access logs.
- Anonymization and De-identification: Anonymizing and de-identifying patient data to protect patient identities while allowing for data analysis and research. This involves removing or masking personally identifiable information (PII) from the data.
- Compliance with Regulations: Ensuring compliance with relevant data protection regulations, such as the Health Insurance Portability and Accountability Act (HIPAA) in the United States and the General Data Protection Regulation (GDPR) in the European Union. Compliance involves implementing policies and procedures that meet regulatory requirements for data privacy and security.
Ethical Implications of Using Patient-Generated Data
The use of patient-generated data raises several ethical considerations that must be addressed to ensure that patients' rights and interests are protected. These considerations include:
- Informed Consent: Obtaining informed consent from patients before collecting and using their data. Patients should be fully informed about the types of data being collected, how it will be used, and their rights to access, correct, and delete their data.
- Transparency: Being transparent with patients about how their data is being used and who has access to it. This includes providing clear and accessible privacy policies and updates on data usage.
- Data Ownership: Recognizing that patients have ownership of their data and the right to control its use. Patients should have the ability to access, manage, and share their data as they see fit.
- Equity and Access: Ensuring that the benefits of using patient-generated data are equitably distributed and that all patients have access to the tools and resources needed to generate and share their data. This involves addressing barriers to access, such as digital literacy and access to technology.
Challenges and Barriers
Technical and Logistical Challenges
Integrating patient-generated data into precision medicine faces several technical and logistical challenges that must be addressed to ensure successful implementation. These challenges include:
- Interoperability: Ensuring that data from various sources can be seamlessly integrated and used together. This requires the use of standardized data formats and protocols, as well as compatible systems and platforms.
- Data Quality: Ensuring the accuracy, reliability, and completeness of patient-generated data. This involves using validated devices and tools, as well as implementing quality control measures to detect and correct errors.
- Scalability: Managing the large volumes of data generated by wearable devices, mobile apps, and other sources. This requires scalable data storage and processing solutions that can handle the increasing amounts of data.
- Integration with Clinical Workflows: Ensuring that patient-generated data can be easily integrated into clinical workflows and used by healthcare providers. This involves designing user-friendly interfaces and tools that support the efficient use of data in clinical practice.
Addressing Patient and Provider Concerns
Both patients and providers may have concerns about the use of patient-generated data that must be addressed to ensure successful adoption. These concerns include:
- Patient Concerns:
- Privacy and Security: Patients may be concerned about the privacy and security of their data. Addressing these concerns involves implementing robust data protection measures and being transparent about data usage.
- Data Ownership and Control: Patients may want to know who owns their data and how it will be used. Ensuring that patients have control over their data and can access, manage, and share it as they see fit is essential.
- Usability and Accessibility: Patients may have concerns about the usability and accessibility of data collection tools. Providing user-friendly and accessible tools, as well as support and training, can help address these concerns.
- Provider Concerns:
- Data Overload: Providers may be concerned about being overwhelmed by the large volumes of patient-generated data. Implementing tools and systems that can filter and prioritize data can help manage this issue.
- Data Integration: Providers may be concerned about the integration of patient-generated data into their clinical workflows. Designing interfaces and tools that support seamless data integration and use can help address this concern.
- Reimbursement: Providers may be concerned about reimbursement for the use of patient-generated data in clinical practice. Advocating for policies and reimbursement models that support the use of PGD can help address this issue.
Case Studies and Applications
Examples of Patient-Generated Data in Practice
Several case studies demonstrate the successful use of patient-generated data in precision medicine, highlighting the potential benefits and outcomes of this approach. Examples include:
- Case Study 1: Diabetes Management: A study conducted by the University of California, San Francisco (UCSF) used continuous glucose monitors (CGMs) to collect real-time blood glucose data from patients with diabetes. The data was integrated with the patients' EHRs and used to tailor insulin doses and lifestyle interventions. The study found that the use of CGMs and personalized treatment plans led to improved glycemic control and reduced risk of complications.
- Case Study 2: Cardiovascular Health: The Cleveland Clinic implemented a remote monitoring program for heart failure patients using wearable devices that tracked vital signs and physical activity. The data was transmitted to healthcare providers in real-time, allowing for timely interventions and adjustments to treatment plans. The program resulted in reduced hospital readmissions and improved patient outcomes.
- Case Study 3: Cancer Care: Mount Sinai Health System used patient-generated data from wearable devices and mobile health apps to monitor symptoms and treatment side effects in cancer patients undergoing chemotherapy. The data was integrated with the patients' EHRs and used to personalize treatment plans and manage side effects. The program improved patient satisfaction and quality of life.
Success Stories and Outcomes
The successful use of patient-generated data in precision medicine has led to several positive outcomes, including:
- Improved Patient Engagement: Patients who actively participate in tracking their health metrics and reporting outcomes are more engaged in their care and more likely to adhere to treatment plans.
- Enhanced Personalized Care: The integration of patient-generated data with clinical data allows for more accurate and personalized treatment plans, leading to better health outcomes.
- Timely Interventions: Real-time monitoring and data analysis enable timely interventions and adjustments to treatment plans, reducing the risk of complications and improving patient outcomes.
- Increased Satisfaction: Patients report higher satisfaction with their care when they are actively involved and can see the impact of their contributions to their health management.
Conclusion
Recap of the Importance of Patient-Generated Data
Patient-generated data is a valuable resource for precision medicine, providing continuous and personalized information that enhances the understanding of individual health. By integrating PGD with clinical data, healthcare providers can develop tailored treatment plans, improve diagnostic accuracy, and achieve better patient outcomes. The use of PGD fosters greater patient engagement and empowers individuals to take an active role in managing their health.
Future Potential and Ongoing Research
The future potential of patient-generated data in precision medicine is vast, with ongoing research and technological advancements poised to further enhance its impact. Emerging trends such as advanced wearable devices, AI-powered data analysis, and improved data integration tools will continue to drive the adoption and effectiveness of PGD. As healthcare organizations address the challenges and ethical considerations associated with PGD, the integration of patient-generated data into clinical practice will become more widespread, leading to a more personalized, efficient, and patient-centered healthcare system.
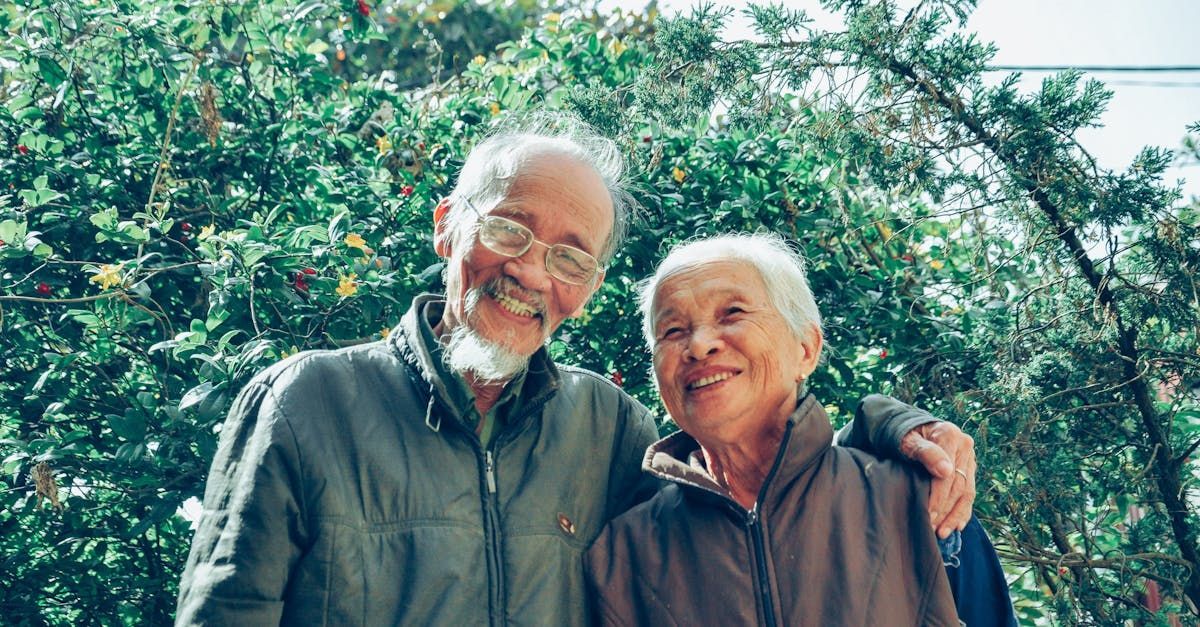
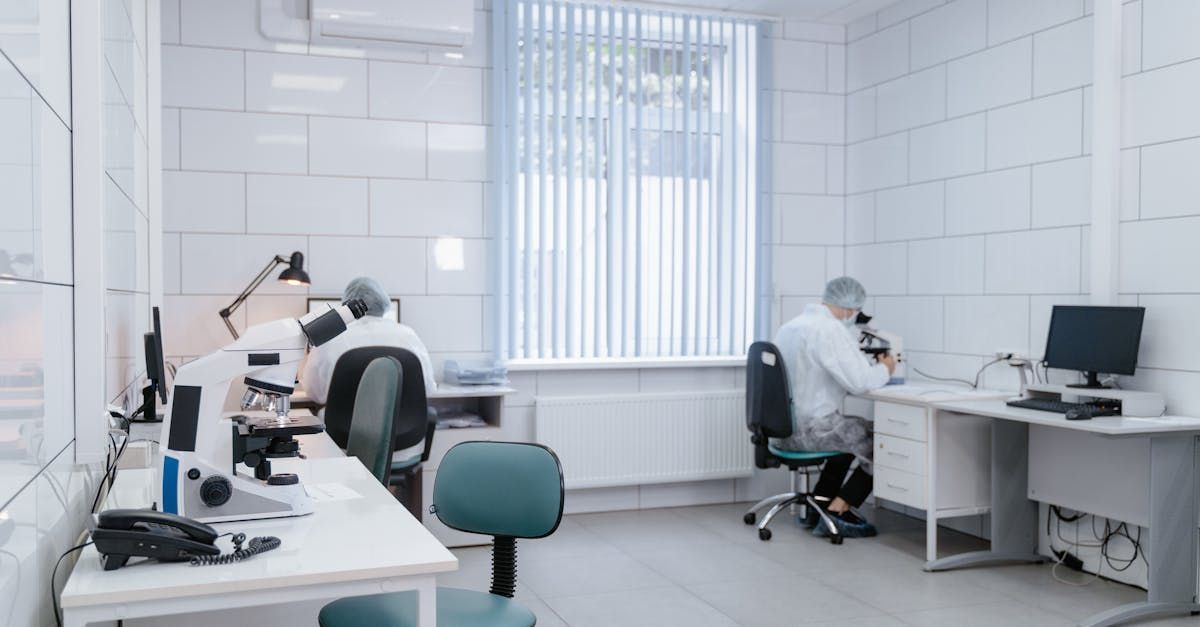