Clinical Decision Support Systems in Precision Medicine
Precision medicine represents a transformative approach in healthcare, tailoring medical treatment to the individual characteristics of each patient. By utilizing genetic, environmental, and lifestyle information, precision medicine enables healthcare providers to develop more effective and targeted therapies. This personalized approach has the potential to improve patient outcomes, reduce adverse effects, and enhance the overall efficiency of healthcare delivery. The significance of precision medicine in modern healthcare cannot be overstated, as it offers a pathway to more accurate diagnoses, optimized treatments, and preventive strategies that are specifically designed for individual patients.
Introduction to Clinical Decision Support Systems (CDSS) and Their Role in Precision Medicine
Clinical Decision Support Systems (CDSS) are pivotal in the implementation of precision medicine. These advanced systems leverage data analytics, machine learning, and artificial intelligence to assist healthcare providers in making informed, evidence-based clinical decisions. By integrating vast amounts of patient data, CDSS can offer personalized recommendations for diagnosis, treatment, and disease management. In the realm of precision medicine, CDSS play a crucial role by providing actionable insights that align with the unique genetic and phenotypic profiles of patients, thus facilitating more precise and effective healthcare interventions.
Understanding Clinical Decision Support Systems
Clinical Decision Support Systems (CDSS) are sophisticated health information technologies designed to provide healthcare professionals with clinical knowledge and patient-specific information, intelligently filtered and presented at appropriate times, to enhance patient care. The main components of CDSS typically include:
- Knowledge Base: This contains the medical knowledge, which may include clinical guidelines, drug databases, and diagnostic criteria.
- Inference Engine: This processes the patient data against the knowledge base to generate recommendations or alerts.
- Communication Mechanism: This presents the processed information to the healthcare provider in an actionable format, such as alerts, reminders, or diagnostic suggestions.
Historical Development and Evolution of CDSS in Healthcare
The evolution of CDSS has been marked by significant milestones that have shaped its current state. Initially, in the 1960s and 1970s, CDSS were rudimentary, often rule-based systems providing simple alerts and reminders. These early systems, such as MYCIN, an expert system for identifying bacterial infections, demonstrated the potential of computer-assisted decision-making in clinical settings.
During the 1980s and 1990s, CDSS evolved with the integration of more complex algorithms and the development of electronic health records (EHRs). This period saw the introduction of more sophisticated systems capable of handling a broader range of clinical scenarios and incorporating more comprehensive patient data.
In the 21st century, advances in computing power, artificial intelligence, and big data analytics have revolutionized CDSS. Modern systems are now capable of real-time data processing, predictive analytics, and personalized recommendations, greatly enhancing their utility in precision medicine.
Types of CDSS: Knowledge-Based, Non-Knowledge-Based, and Hybrid Systems
- Knowledge-Based Systems: These systems rely on a structured knowledge base, often derived from clinical guidelines and expert rules. They use if-then rules to provide recommendations. Examples include drug interaction alerts and clinical guideline reminders.
- Non-Knowledge-Based Systems: These leverage machine learning and artificial intelligence to analyze large datasets and identify patterns. They do not rely on pre-defined rules but instead learn from historical data to predict outcomes and suggest interventions. Examples include predictive modeling for patient outcomes and machine learning algorithms for image analysis.
- Hybrid Systems: Combining elements of both knowledge-based and non-knowledge-based systems, hybrid CDSS integrate structured clinical knowledge with data-driven insights. These systems aim to leverage the strengths of both approaches, providing robust and versatile decision support. An example is a system that uses AI to analyze patient data and then applies clinical guidelines to generate recommendations.
The ongoing development and refinement of CDSS are crucial in advancing precision medicine, as these systems enhance the ability of healthcare providers to deliver personalized, data-driven care tailored to the unique needs of each patient.
Integration of CDSS in Precision Medicine
How CDSS Supports Precision Medicine by Providing Personalized Treatment Recommendations
Clinical Decision Support Systems (CDSS) are integral to the advancement of precision medicine by enabling the delivery of highly personalized treatment recommendations. By incorporating vast and complex datasets, CDSS can analyze individual patient data to provide customized clinical guidance. These systems enhance the precision of medical decisions in several ways:
- Tailored Treatment Plans: CDSS can recommend specific therapies based on an individual’s genetic makeup, disease profile, and personal health data. This reduces the trial-and-error approach often seen in conventional treatments and increases the likelihood of successful outcomes.
- Predictive Analytics: Using historical data and machine learning algorithms, CDSS can predict disease progression and treatment responses, helping clinicians to choose the most effective interventions for each patient.
- Risk Stratification: CDSS can identify patients at high risk of developing certain conditions based on their genetic predispositions and lifestyle factors, enabling early intervention and preventive care.
Role of Genetic Data, Biomarkers, and Patient History in CDSS
The effectiveness of CDSS in precision medicine heavily relies on the integration of various types of data, including genetic information, biomarkers, and comprehensive patient histories:
- Genetic Data: By incorporating genetic information, CDSS can identify specific genetic mutations that influence disease risk and treatment response. For example, CDSS can recommend targeted therapies for cancer patients based on their tumor’s genetic profile.
- Biomarkers: Biomarkers, which are measurable indicators of a biological condition, play a crucial role in precision medicine. CDSS can analyze biomarker levels to provide insights into disease activity and treatment efficacy, facilitating timely adjustments to therapy plans.
- Patient History: A thorough understanding of a patient’s medical history, including past illnesses, treatments, and outcomes, is essential for making informed clinical decisions. CDSS use this historical data to identify patterns and predict future health events, contributing to more personalized and effective care.
Case Studies Demonstrating the Successful Integration of CDSS in Clinical Practice
- Oncology: One notable example is the use of CDSS in oncology to guide the treatment of cancer patients. Systems like IBM Watson for Oncology analyze vast amounts of data, including patient records, clinical guidelines, and medical literature, to provide oncologists with evidence-based treatment options tailored to the genetic profile of the patient’s tumor. This approach has led to improved treatment outcomes and reduced side effects.
- Cardiology: In cardiology, CDSS have been used to manage patients with heart disease by integrating genetic data and biomarkers to predict the risk of cardiovascular events. For instance, a CDSS can help identify patients who are at high risk of a heart attack based on their genetic predisposition and cholesterol levels, enabling preemptive measures such as lifestyle changes and medication adjustments.
- Diabetes Management: In the management of diabetes, CDSS can combine patient history, genetic information, and real-time glucose monitoring data to provide personalized insulin dosing recommendations. This has been shown to improve glycemic control and reduce the risk of complications associated with diabetes.
The integration of CDSS in precision medicine exemplifies how technology can enhance clinical decision-making, leading to more precise, effective, and personalized healthcare. By leveraging the power of data, CDSS enable healthcare providers to deliver treatments that are tailored to the unique needs of each patient, ultimately improving outcomes and quality of life.
Benefits of CDSS in Precision Medicine
Improved Accuracy and Efficiency in Diagnosis and Treatment Planning
One of the foremost benefits of Clinical Decision Support Systems (CDSS) in precision medicine is the significant enhancement in the accuracy and efficiency of diagnosis and treatment planning. CDSS can analyze vast amounts of patient data, including genetic information, biomarkers, and medical histories, to provide precise and timely recommendations. This capability reduces the time healthcare providers spend on diagnosing conditions and developing treatment plans. Additionally, the integration of CDSS helps in identifying the most effective therapies based on individual patient profiles, thereby optimizing treatment strategies and reducing the likelihood of ineffective or harmful interventions.
Enhanced Patient Outcomes Through Personalized Care
CDSS facilitate the delivery of highly personalized care, which is central to the ethos of precision medicine. By tailoring treatments to the unique genetic and phenotypic characteristics of each patient, CDSS can significantly improve patient outcomes. For instance, in oncology, CDSS can recommend targeted therapies that are more likely to be effective based on the genetic mutations present in a patient’s tumor. This approach not only improves the efficacy of treatments but also minimizes side effects and enhances the overall quality of life for patients. Furthermore, by continuously monitoring patient data, CDSS can provide ongoing adjustments to treatment plans, ensuring that patients receive the most appropriate and up-to-date care.
Reduction of Medical Errors and Improved Adherence to Clinical Guidelines
Medical errors are a significant concern in healthcare, often leading to adverse patient outcomes and increased healthcare costs. CDSS play a crucial role in mitigating these errors by providing evidence-based recommendations and real-time alerts to healthcare providers. For example, CDSS can alert clinicians to potential drug interactions, allergies, and contraindications, thereby preventing harmful prescribing errors. Additionally, CDSS promote adherence to clinical guidelines by integrating the latest medical research and protocols into their knowledge base. This ensures that healthcare providers consistently follow best practices, leading to standardized and high-quality care across different clinical settings.
The benefits of CDSS in precision medicine are multifaceted, encompassing improvements in diagnostic accuracy, treatment efficiency, patient outcomes, and the reduction of medical errors. As these systems continue to evolve and integrate more advanced technologies, their impact on precision medicine will likely grow, further transforming the landscape of modern healthcare.
Challenges and Limitations
Technical Challenges in Implementing and Maintaining CDSS
Implementing and maintaining Clinical Decision Support Systems (CDSS) presents several technical challenges. One of the primary issues is the integration of CDSS with existing Electronic Health Records (EHR) systems. Many healthcare institutions use a variety of EHR systems, which may not be compatible with each other or with new CDSS platforms. This lack of standardization can make it difficult to implement CDSS effectively.
Additionally, maintaining CDSS requires regular updates to ensure the system incorporates the latest clinical guidelines, medical research, and technological advancements. This ongoing maintenance can be resource-intensive, requiring continuous input from IT specialists, clinicians, and researchers. Moreover, ensuring the accuracy and reliability of the data processed by CDSS is critical, as errors in data integration or analysis can lead to incorrect recommendations, potentially harming patients.
Data Privacy and Security Concerns
Data privacy and security are significant concerns when implementing CDSS, particularly given the sensitive nature of health data. The integration of CDSS involves the collection, storage, and analysis of vast amounts of patient information, including genetic data, which is highly personal and sensitive. Ensuring this data is protected from breaches and unauthorized access is paramount.
Healthcare institutions must comply with stringent data protection regulations, such as the Health Insurance Portability and Accountability Act (HIPAA) in the United States or the General Data Protection Regulation (GDPR) in Europe. Implementing robust cybersecurity measures is essential to safeguard patient data. However, achieving this level of security can be challenging and costly, requiring ongoing investment in cybersecurity infrastructure and training for healthcare personnel.
The Need for Interoperability Between Different Healthcare Systems and Platforms
Interoperability, or the ability of different healthcare systems and platforms to work together seamlessly, is crucial for the effective implementation of CDSS. Many healthcare providers operate using disparate systems that do not communicate well with each other. This lack of interoperability can hinder the ability of CDSS to access and integrate comprehensive patient data, limiting their effectiveness.
Achieving interoperability requires standardizing data formats and protocols across different healthcare systems. Efforts such as the Fast Healthcare Interoperability Resources (FHIR) standard aim to address these issues by providing a framework for exchanging healthcare information electronically. However, widespread adoption of these standards is still a work in progress, and achieving full interoperability remains a significant challenge.
Emerging Technologies and Innovations
The Role of Artificial Intelligence and Machine Learning in Enhancing CDSS Capabilities
Artificial intelligence (AI) and machine learning (ML) are at the forefront of technological advancements enhancing Clinical Decision Support Systems (CDSS). These technologies enable CDSS to analyze large volumes of complex healthcare data more efficiently and accurately than traditional methods. AI algorithms can identify patterns and correlations within patient data that may not be evident to human clinicians, leading to more precise diagnostics and personalized treatment recommendations.
Machine learning, a subset of AI, allows CDSS to continuously learn and improve from new data. As ML algorithms are exposed to more patient data and clinical outcomes, their predictive accuracy and decision-making capabilities are refined. For example, in oncology, ML models can predict patient responses to various treatment protocols based on genetic profiles and historical outcomes, leading to more tailored and effective treatment plans.
Advances in Natural Language Processing and Their Impact on CDSS
Natural Language Processing (NLP) is another critical technology driving the evolution of CDSS. NLP enables CDSS to understand and interpret unstructured data from various sources, such as clinical notes, research papers, and patient narratives. By converting this unstructured data into structured, actionable insights, NLP enhances the decision-making capabilities of CDSS.
For instance, NLP can analyze physician's notes and extract relevant information about a patient's symptoms, medical history, and treatment responses. This information can then be integrated with structured data from electronic health records (EHRs) to provide a comprehensive view of the patient's health status. Consequently, NLP-equipped CDSS can offer more holistic and accurate clinical recommendations, improving patient care outcomes.
Integration of Big Data Analytics in CDSS
Big data analytics is transforming the healthcare landscape by enabling the integration and analysis of massive datasets from diverse sources. In the context of CDSS, big data analytics can synthesize information from EHRs, genomic databases, wearable devices, and population health records to provide a more comprehensive and nuanced understanding of patient health.
The integration of big data analytics in CDSS allows for advanced predictive modeling and risk stratification. For example, by analyzing data from millions of patients, CDSS can identify risk factors for chronic diseases, predict disease outbreaks, and recommend preventive measures tailored to individual patients. This data-driven approach ensures that clinical decisions are based on the most extensive and up-to-date information available, enhancing the precision and effectiveness of medical interventions.
Case Studies and Real-World Applications
Detailed Case Studies Showcasing Successful Use of CDSS in Various Medical Fields
- Oncology: In oncology, CDSS has revolutionized treatment planning and patient care. For example, IBM Watson for Oncology uses AI to analyze patient data, medical literature, and clinical trial results to provide oncologists with evidence-based treatment options. At Memorial Sloan Kettering Cancer Center, Watson has been used to match patients with appropriate clinical trials, significantly increasing trial enrollment and improving treatment outcomes.
- Cardiology: The integration of CDSS in cardiology has led to improved management of heart diseases. The University of California, San Francisco (UCSF) implemented a CDSS to analyze patient data for early detection of heart failure. By identifying high-risk patients through predictive analytics, the system enabled timely interventions that reduced hospital readmissions and improved patient prognosis.
- Diabetes Management: The Mayo Clinic utilized a CDSS to enhance diabetes care by providing personalized insulin dosing recommendations based on continuous glucose monitoring data. This system improved glycemic control and reduced the incidence of hypoglycemia, demonstrating the potential of CDSS to optimize chronic disease management.
- Notable Labs: Notable Labs has been at the forefront of leveraging CDSS to advance precision medicine. By integrating CDSS with their proprietary data analytics platform, Notable Labs can analyze patient-specific genetic and phenotypic data to identify the most effective treatments for various conditions, particularly in oncology. This approach has led to significant improvements in patient outcomes and has demonstrated the value of personalized treatment strategies.
Lessons Learned from Real-World Implementations
- Integration with Existing Systems: Successful implementation of CDSS requires seamless integration with existing electronic health records (EHR) and other healthcare systems. This ensures that CDSS can access comprehensive patient data and provide accurate recommendations. Healthcare providers have learned that collaboration between IT teams, clinicians, and CDSS vendors is crucial for achieving this integration.
- User Training and Engagement: One of the key lessons from real-world implementations is the importance of user training and engagement. Clinicians need to understand how to use CDSS effectively and trust the system’s recommendations. Regular training sessions and continuous feedback loops have been essential in building this trust and ensuring widespread adoption of CDSS.
- Data Quality and Standardization: The accuracy and effectiveness of CDSS depend heavily on the quality and standardization of the data they analyze. Ensuring high-quality data entry and maintaining standardized data formats across different healthcare systems have been critical lessons for optimizing CDSS performance.
Patient and Clinician Perspectives on CDSS
- Patient Perspectives: From the patient’s viewpoint, CDSS can enhance the quality of care by providing more accurate diagnoses and personalized treatment plans. Patients have reported higher satisfaction levels when their treatments are tailored to their specific needs, reducing the trial-and-error approach often seen in traditional medicine. Additionally, CDSS can empower patients by providing them with more information about their conditions and treatment options.
- Clinician Perspectives: Clinicians generally view CDSS as valuable tools that augment their expertise and improve clinical decision-making. By providing evidence-based recommendations and real-time alerts, CDSS can help reduce cognitive overload and prevent medical errors. However, some clinicians may initially be skeptical about relying on automated systems. Addressing concerns about the accuracy and reliability of CDSS through training and transparent communication is essential for gaining clinician buy-in.
Future Directions and Research
Potential Advancements in CDSS and Their Impact on the Future of Precision Medicine
The future of Clinical Decision Support Systems (CDSS) in precision medicine is promising, with several potential advancements on the horizon:
- Advanced AI and Deep Learning Algorithms: The integration of more sophisticated artificial intelligence (AI) and deep learning algorithms will enhance the predictive capabilities of CDSS. These advancements will allow CDSS to process and analyze even more complex datasets, leading to more accurate and personalized treatment recommendations.
- Genomic and Multi-Omics Data Integration: Future CDSS will increasingly incorporate genomic, proteomic, metabolomic, and other omics data to provide a holistic view of a patient's health. This comprehensive approach will enable the identification of novel biomarkers and therapeutic targets, further personalizing and refining treatment plans.
- Real-Time Data Processing and Feedback: The ability to process and analyze data in real-time will become a standard feature of CDSS. This will allow for immediate adjustments to treatment plans based on the latest patient data, improving the responsiveness and effectiveness of medical interventions.
- Enhanced User Interfaces and Experience: Future CDSS will focus on improving user interfaces and overall user experience. By making these systems more intuitive and easier to use, healthcare providers will be able to integrate CDSS more seamlessly into their workflows, increasing adoption and utilization.
Ongoing Research and Development Efforts in CDSS
Several ongoing research and development efforts are focused on advancing CDSS:
- Interdisciplinary Research Initiatives: Collaborative research initiatives that bring together experts from various fields, including computer science, medicine, and bioinformatics, are driving innovations in CDSS. These interdisciplinary efforts aim to develop more robust and versatile systems capable of handling diverse medical scenarios.
- Clinical Trials and Validation Studies: Numerous clinical trials and validation studies are being conducted to assess the efficacy and safety of CDSS in different medical fields. These studies provide critical evidence to support the broader adoption of CDSS and help identify areas for improvement.
- Integration with Emerging Technologies: Research is ongoing to integrate CDSS with emerging technologies such as wearable devices, Internet of Things (IoT), and blockchain. These integrations aim to enhance data collection, security, and interoperability, further improving the functionality and reliability of CDSS.
Collaboration Between Technology Developers, Healthcare Providers, and Researchers
Collaboration is essential for the continued advancement and successful implementation of CDSS:
- Technology Developers: Technology developers play a crucial role in creating and refining CDSS. By working closely with healthcare providers and researchers, they can ensure that these systems meet the practical needs of clinical settings and incorporate the latest scientific advancements.
- Healthcare Providers: Healthcare providers are integral to the development and deployment of CDSS. Their insights and feedback are invaluable in designing systems that are user-friendly and effective in real-world scenarios. Collaboration with technology developers helps tailor CDSS to specific clinical workflows and patient care protocols.
- Researchers: Researchers contribute by providing the scientific foundation for CDSS. Their work on understanding diseases, identifying biomarkers, and developing predictive models is critical for the continuous improvement of these systems. Collaboration with technology developers ensures that the latest research findings are quickly translated into practical applications.
- Notable Labs: As a leader in precision medicine, Notable Labs exemplifies the importance of collaboration in advancing CDSS. By partnering with academic institutions, healthcare providers, and technology developers, Notable Labs is at the forefront of integrating cutting-edge technologies into their CDSS platforms, driving innovation and improving patient outcomes.
Recap of the importance of CDSS in precision medicine.
Clinical Decision Support Systems (CDSS) are pivotal in advancing precision medicine by providing healthcare professionals with tools to make more accurate, data-driven decisions tailored to individual patient needs. Through the integration of vast and diverse datasets, CDSS enable personalized treatment plans that enhance patient outcomes, improve diagnostic accuracy, and streamline clinical workflows. By incorporating genetic information, biomarkers, and comprehensive patient histories, CDSS deliver personalized recommendations that go beyond traditional one-size-fits-all approaches, making precision medicine a reality.
The transformative potential of CDSS in healthcare delivery is immense. As these systems continue to evolve, their impact will be felt across various aspects of healthcare:
- Enhanced Patient Care: By providing personalized treatment recommendations, CDSS can significantly improve patient care, leading to better outcomes and higher patient satisfaction. Tailored treatment plans reduce the likelihood of adverse reactions and improve the efficacy of interventions.
- Efficiency and Cost-Effectiveness: CDSS streamline the diagnostic and treatment planning processes, reducing the time and resources required for effective patient care. This increased efficiency can lead to cost savings for healthcare providers and patients alike.
- Reduction of Medical Errors: CDSS provide real-time alerts and evidence-based recommendations that help prevent medical errors, such as incorrect drug prescriptions or missed diagnoses. This improves patient safety and reduces the incidence of preventable complications.
- Integration of Emerging Technologies: The integration of AI, machine learning, natural language processing, and big data analytics will continue to enhance the capabilities of CDSS, making them even more powerful tools in precision medicine. These advancements will enable CDSS to process more complex data and provide even more accurate and timely recommendations.
The continued research and development of CDSS are crucial for realizing their full potential in transforming healthcare. Stakeholders across the healthcare ecosystem—including technology developers, healthcare providers, researchers, and policymakers—must work collaboratively to address current challenges and drive innovation in this field. Key areas for ongoing focus include:
- Interdisciplinary Collaboration: Encouraging partnerships between clinicians, researchers, and technology developers will ensure that CDSS are designed to meet the practical needs of healthcare settings and incorporate the latest scientific advancements.
- Investment in Emerging Technologies: Continued investment in AI, machine learning, and other emerging technologies will enhance the predictive and analytical capabilities of CDSS, making them more effective in supporting precision medicine.
- Focus on Data Quality and Security: Ensuring high-quality, standardized data and robust security measures will be critical in maintaining the accuracy and reliability of CDSS while protecting patient privacy.
- Education and Training: Providing education and training for healthcare providers on the use of CDSS will promote widespread adoption and effective utilization of these systems, maximizing their impact on patient care.
Clinical Decision Support Systems (CDSS) are integral to the future of precision medicine, offering significant benefits in terms of accuracy, efficiency, and personalized patient care. The potential of CDSS to transform healthcare delivery is vast, but realizing this potential requires continued research, development, and collaboration among all stakeholders. By addressing current challenges and investing in emerging technologies, we can ensure that CDSS remain at the forefront of precision medicine, ultimately improving healthcare outcomes for patients worldwide.
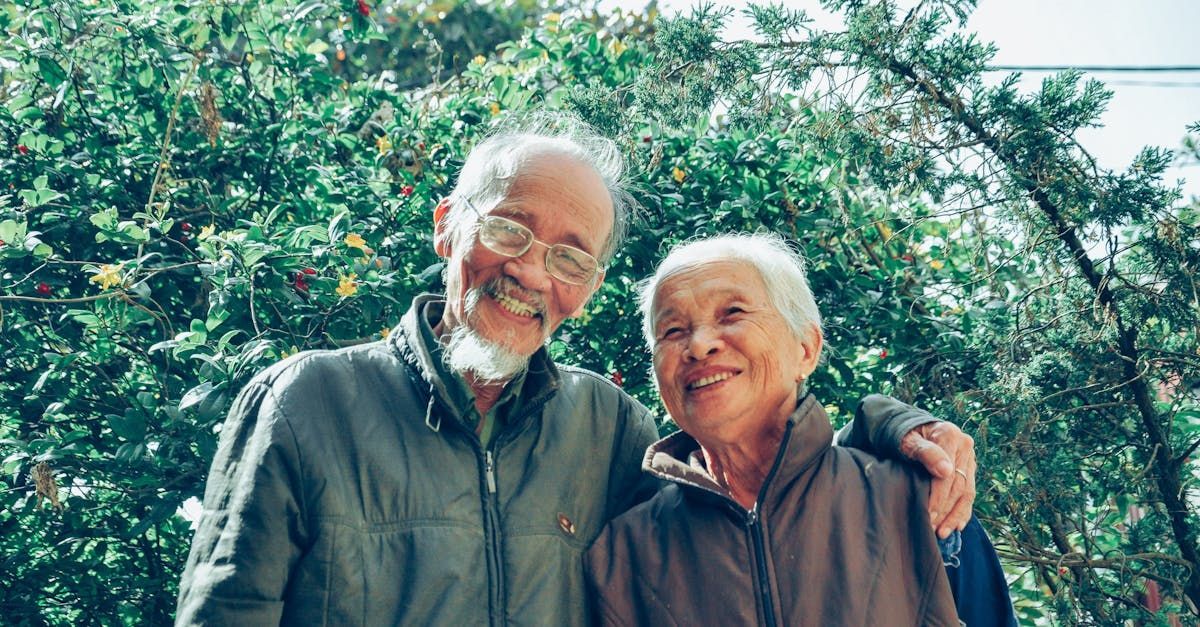
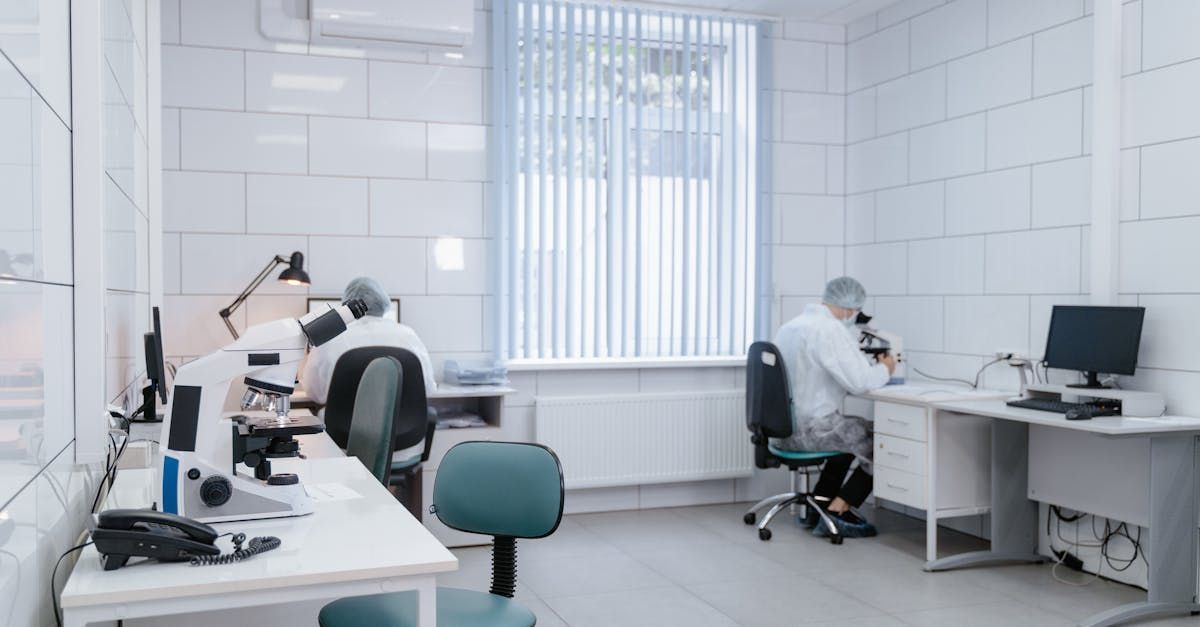