AI and Machine Learning in Precision Medicine
Precision medicine is revolutionizing the healthcare industry by tailoring treatments to individual patients based on their genetic makeup, environment, and lifestyle. This personalized approach aims to improve treatment efficacy and reduce adverse effects, leading to better patient outcomes. Precision medicine contrasts sharply with the traditional one-size-fits-all approach, providing customized healthcare solutions that cater specifically to each patient's unique characteristics.
Artificial intelligence (AI) and machine learning (ML) are at the forefront of this revolution, offering powerful tools to analyze vast amounts of data quickly and accurately. AI and ML can identify patterns and correlations in data that would be impossible for humans to discern, driving innovations in diagnosis, treatment planning, and drug development. These technologies are enhancing the precision and effectiveness of medical care, making it possible to predict patient responses to treatments and tailor interventions more accurately than ever before.
The purpose of this blog is to explore how AI and machine learning are enhancing precision medicine techniques. We will delve into various applications of these technologies, including data analysis, diagnostic accuracy, personalized treatment plans, drug discovery, real-time monitoring, and adaptive treatment. Additionally, we will discuss the challenges and ethical considerations associated with integrating AI and ML into healthcare, as well as future directions and innovations in this exciting field.
Understanding AI and Machine Learning
Definition of AI and Machine Learning
Artificial intelligence (AI) refers to the simulation of human intelligence in machines that are programmed to think and learn. AI systems can perform tasks that typically require human intelligence, such as visual perception, speech recognition, decision-making, and language translation.
Machine learning (ML), a subset of AI, involves the use of algorithms and statistical models to enable machines to improve their performance on a task through experience. Rather than being explicitly programmed to perform a task, ML systems learn from data, identifying patterns and making predictions based on that data.
Key Concepts and Technologies in AI and Machine Learning
- Neural Networks: Modeled after the human brain, neural networks consist of interconnected nodes (neurons) that process and transmit information. They are used in deep learning to recognize patterns and make complex decisions.
- Deep Learning: A subset of ML that uses neural networks with many layers (hence "deep") to analyze various levels of data. Deep learning is particularly effective for image and speech recognition, as well as other complex tasks.
- Natural Language Processing (NLP): This technology enables machines to understand, interpret, and respond to human language. In healthcare, NLP can be used to analyze clinical notes and research papers.
- Supervised Learning: A type of ML where the model is trained on labeled data. It is used to predict outcomes based on input-output pairs, making it useful for tasks like disease diagnosis.
- Unsupervised Learning: Involves training a model on unlabeled data, allowing it to identify patterns and relationships. This can be used for clustering patients with similar health profiles.
- Reinforcement Learning: A type of ML where an agent learns by interacting with its environment, receiving rewards for desirable actions. It can optimize treatment plans by continuously improving based on feedback.
Overview of How These Technologies Are Applied in Healthcare
AI and machine learning are transforming healthcare by enabling more precise and efficient medical practices. They are applied in various ways, including:
- Data Analysis: AI algorithms can process large datasets quickly, identifying trends and patterns that can inform medical decisions. For instance, they can analyze genetic information to predict disease risk.
- Diagnostic Accuracy: Machine learning models can improve diagnostic accuracy by interpreting medical images, such as X-rays and MRIs, more accurately than human radiologists in some cases.
- Personalized Treatment Plans: AI can help develop personalized treatment plans by analyzing a patient's genetic makeup, lifestyle, and other factors, predicting which treatments are likely to be most effective.
- Drug Discovery: Machine learning accelerates drug discovery by predicting how different compounds will interact with biological targets, thus reducing the time and cost involved in bringing new drugs to market.
- Real-Time Monitoring and Predictive Analytics: Wearable devices and sensors can collect real-time health data, which AI can analyze to detect early signs of disease or predict health events, such as heart attacks.
- Adaptive Treatment: AI systems can continuously learn from patient data, adapting treatment plans as more information becomes available, ensuring that patients receive the most effective care possible.
By integrating AI and machine learning into healthcare, we are moving towards a future where medical care is not only more efficient but also more tailored to individual patient needs, leading to better outcomes and enhanced quality of life.
AI and Machine Learning in Data Analysis
Role of AI in Processing and Analyzing Large Datasets
In the field of precision medicine, the ability to process and analyze vast amounts of data is crucial. AI plays a pivotal role in this process by leveraging its computational power to handle and interpret large datasets efficiently. Traditional methods of data analysis are often time-consuming and prone to human error, whereas AI-driven approaches can quickly sift through complex data, providing insights that might otherwise remain hidden.
Notable Labs is at the forefront of utilizing AI and machine learning in precision medicine through its Predictive Precision Medicine Platform (PPMP). This platform integrates advanced computational algorithms with biological and clinical data to accurately predict patient responses to various treatments. By employing high-throughput automated processes and leveraging a vast data repository containing billions of data points from patient samples, Notable Labs’ PPMP identifies and selects patients who are most likely to respond to specific therapies. This approach not only enhances the precision of treatment but also fast-tracks the clinical development of promising therapeutic candidates
AI algorithms are designed to manage various types of healthcare data, including:
- Genomic Data: Sequencing entire genomes generates massive amounts of data. AI can process this data to identify genetic variations and mutations linked to specific diseases.
- Clinical Data: Patient records, including lab results, imaging studies, and clinical notes, contain valuable information. AI can integrate and analyze this data to identify trends and predict outcomes.
- Sensor Data: Wearable devices and health monitoring systems generate continuous streams of data. AI can analyze this real-time data to detect anomalies and predict potential health issues.
How Machine Learning Algorithms Identify Patterns and Correlations
Machine learning algorithms excel at identifying patterns and correlations within data. These algorithms can learn from historical data and apply this knowledge to new, unseen data, making them highly effective for predictive analytics. Here are some key techniques used in machine learning for data analysis:
- Supervised Learning: In supervised learning, algorithms are trained on labeled datasets, where the outcomes are known. The model learns to map input data to the correct output, making it useful for tasks like predicting disease risk based on genetic markers.
- Unsupervised Learning: Unsupervised learning algorithms work with unlabeled data. They identify inherent structures and patterns within the data, such as grouping patients with similar health profiles, which can be crucial for personalized treatment plans.
- Reinforcement Learning: This approach involves training an algorithm to make decisions through trial and error, receiving feedback in the form of rewards or penalties. It is particularly useful for optimizing treatment strategies over time.
- Deep Learning: Deep learning, a subset of machine learning, uses neural networks with multiple layers to analyze data. It is particularly powerful in recognizing patterns in complex data, such as medical images or genetic sequences.
Examples of AI-Driven Data Analysis in Genomics and Patient Records
- Genomic Data Analysis: AI algorithms can analyze genomic data to identify mutations and variations associated with diseases. For example, deep learning models can pinpoint specific genetic markers linked to cancer, enabling early diagnosis and targeted therapy.
- Predictive Analytics in Patient Records: By analyzing electronic health records (EHRs), machine learning models can predict patient outcomes, such as the likelihood of readmission or the development of complications. These predictions help clinicians intervene early and tailor treatments to individual patients.
- Personalized Treatment Plans: AI can integrate various data sources, including genetic, clinical, and lifestyle data, to create comprehensive patient profiles. Machine learning models then use these profiles to recommend personalized treatment plans, improving efficacy and reducing adverse effects.
- Drug Response Prediction: AI-driven analysis of patient data can predict how individuals will respond to specific drugs. This approach is used in precision oncology, where AI models analyze tumor genomics to recommend the most effective treatment options.
By harnessing the power of AI and machine learning in data analysis, precision medicine is transforming healthcare. These technologies enable more accurate diagnoses, personalized treatment plans, and improved patient outcomes, paving the way for a new era of medical care tailored to individual needs.
Enhancing Diagnostic Accuracy
AI in Medical Imaging and Diagnostics
Artificial Intelligence (AI) is revolutionizing medical imaging and diagnostics by providing tools that enhance the accuracy and efficiency of disease detection and diagnosis. AI algorithms can process vast amounts of imaging data quickly, identifying patterns and anomalies that may be difficult for human eyes to detect. This capability is particularly valuable in radiology and pathology, where timely and accurate interpretation of images is critical for patient outcomes.
Examples: AI Algorithms in Radiology and Pathology
- Radiology:
- AI-Powered Imaging Analysis: AI algorithms can analyze X-rays, CT scans, MRI scans, and other imaging modalities to detect abnormalities such as tumors, fractures, and infections. For instance, AI systems can highlight areas of concern in mammograms, aiding radiologists in identifying early signs of breast cancer.
- Automated Diagnosis: Tools like Google’s DeepMind have developed AI systems that can diagnose over 50 different eye diseases by analyzing retinal scans. These systems can operate with accuracy comparable to that of experienced specialists.
- Pathology:
- Digital Pathology: AI algorithms can analyze high-resolution images of tissue samples to identify cancerous cells. These systems assist pathologists by providing detailed assessments of tissue architecture and cellular features, leading to more accurate diagnoses.
- Histopathological Analysis: AI tools can classify different types of cancer cells and predict tumor grades, helping pathologists determine the aggressiveness of cancers and guide treatment decisions.
Role of AI in Early Disease Detection and Prediction
Early detection of diseases significantly improves treatment outcomes and survival rates. AI excels in identifying early signs of disease by analyzing subtle changes in imaging and clinical data that might be overlooked by human observers. This predictive capability is transforming preventive medicine and enabling proactive healthcare.
Case Studies: Predictive Analytics for Cancer and Cardiovascular Diseases
- Predictive Analytics for Cancer:
- Breast Cancer Detection: AI algorithms have been developed to analyze mammograms and predict the likelihood of breast cancer years before it becomes detectable through conventional methods. These models use historical imaging data to learn patterns associated with early cancer development.
- Lung Cancer Screening: AI systems are being used to analyze low-dose CT scans for early detection of lung cancer. Studies have shown that these systems can reduce false positives and improve the accuracy of nodule detection, leading to earlier interventions and better patient outcomes.
- Cardiovascular Diseases:
- Heart Disease Prediction: AI models can analyze electronic health records (EHRs), including data on cholesterol levels, blood pressure, and lifestyle factors, to predict the risk of heart disease. These models help identify high-risk individuals who may benefit from preventive measures.
- Arrhythmia Detection: Wearable devices equipped with AI algorithms can continuously monitor heart rhythms and detect irregularities indicative of arrhythmias. Early detection of such conditions enables timely treatment and reduces the risk of complications such as stroke.
By enhancing diagnostic accuracy and enabling early disease detection, AI is playing a crucial role in precision medicine. These advancements lead to better patient outcomes, as conditions are identified and treated earlier, and treatments are tailored to the specific needs of each patient. The integration of AI in diagnostics represents a significant step forward in the quest for personalized healthcare, offering hope for more effective management of diseases and improved quality of life for patients.
Personalized Treatment Plans
How AI Tailors Treatment Plans Based on Individual Patient Data
Artificial Intelligence (AI) tailors treatment plans by leveraging vast amounts of individual patient data, including genetic information, medical history, lifestyle factors, and real-time health monitoring data. AI systems analyze this comprehensive data to identify patterns and predict the most effective treatment strategies for each patient. This approach ensures that treatments are customized to meet the unique needs and characteristics of each patient, leading to improved outcomes and reduced adverse effects.
Machine Learning Models Predicting Patient Response to Therapies
Machine learning models are capable of predicting how patients will respond to various therapies by analyzing historical data and identifying correlations between patient characteristics and treatment outcomes. These models consider a multitude of factors, such as genetic mutations, biomarkers, and previous treatment responses, to forecast the efficacy and potential side effects of different treatment options.
- Genetic Profiling: AI algorithms analyze genetic data to identify mutations that may influence treatment response. For example, in oncology, certain genetic mutations are associated with resistance or sensitivity to specific chemotherapy drugs or targeted therapies.
- Biomarker Analysis: Machine learning models can detect biomarkers in patient samples that predict how well a patient will respond to a particular treatment. This allows for the selection of the most appropriate therapy based on the patient's biomarker profile.
- Real-Time Health Monitoring: Wearable devices and other health monitoring technologies provide continuous data on patient health. AI systems use this data to adjust treatment plans in real-time, ensuring that interventions are responsive to changes in the patient's condition.
Case Studies: AI-Driven Personalized Medicine in Oncology and Chronic Diseases
- Oncology:
- Breast Cancer Treatment: AI-driven platforms analyze genetic data and tumor characteristics to recommend personalized treatment plans for breast cancer patients. For instance, AI models can predict which patients are likely to benefit from hormone therapy versus chemotherapy, reducing unnecessary treatments and improving outcomes.
- Lung Cancer Management: AI algorithms can assess the genetic profile of lung cancer tumors to identify the most effective targeted therapies. By analyzing data from clinical trials and patient outcomes, AI models help oncologists make informed decisions about treatment options, leading to better survival rates and quality of life for patients.
- Chronic Diseases:
- Diabetes Management: AI systems analyze data from continuous glucose monitors, insulin pumps, and patient lifestyle factors to create personalized insulin dosing recommendations. These systems adapt to the patient's unique glucose patterns and lifestyle, optimizing blood sugar control and reducing the risk of complications.
- Cardiovascular Disease: AI models predict the risk of adverse cardiovascular events by analyzing patient data, including cholesterol levels, blood pressure, and genetic markers. Personalized treatment plans are then developed to manage risk factors and prevent heart attacks or strokes.
By tailoring treatment plans to the individual needs of patients, AI-driven personalized medicine significantly improves the effectiveness of treatments and enhances patient outcomes. The ability to predict patient responses to therapies allows for more precise and targeted interventions, reducing the trial-and-error approach traditionally associated with medical treatments. As AI continues to evolve, its application in personalized medicine holds great promise for transforming healthcare and delivering more effective, patient-centered care.
Drug Discovery and Development
AI Accelerating the Drug Discovery Process
Artificial Intelligence (AI) is revolutionizing the drug discovery process by significantly reducing the time and cost required to develop new medications. Traditionally, drug discovery is a lengthy and expensive endeavor, often taking over a decade and billions of dollars to bring a new drug to market. AI addresses these challenges by automating and optimizing various stages of the drug discovery pipeline.
Examples: Virtual Screening and Drug Repurposing
- Virtual Screening:
- AI-powered virtual screening involves using machine learning algorithms to analyze large libraries of chemical compounds to identify potential drug candidates. These algorithms predict how different compounds will interact with specific biological targets, such as proteins or enzymes involved in a disease process.
- By rapidly screening millions of compounds, AI can identify promising candidates that are most likely to succeed in further testing, significantly reducing the initial phase of drug discovery.
- Drug Repurposing:
- AI is also used to identify new therapeutic uses for existing drugs, a process known as drug repurposing. Machine learning models analyze extensive datasets, including clinical trial results, patient records, and scientific literature, to find correlations between existing drugs and new disease targets.
- Drug repurposing is advantageous because it bypasses many early-stage development hurdles, allowing for faster and more cost-effective entry into clinical trials.
Role of Machine Learning in Predicting Drug Efficacy and Safety
Machine learning models play a crucial role in predicting the efficacy and safety of potential drug candidates. By analyzing historical data from clinical trials, preclinical studies, and real-world patient outcomes, these models can identify patterns that indicate how a drug will perform in humans.
- Predicting Drug Efficacy:
- Machine learning algorithms assess the likelihood that a drug will achieve its intended therapeutic effect by analyzing genetic, proteomic, and metabolic data. These predictions help prioritize compounds that have the highest potential for success in clinical trials.
- By simulating how drugs interact with biological systems, AI models can identify the optimal dosage and administration routes, enhancing the overall efficacy of the treatment.
- Ensuring Drug Safety:
- Safety is a paramount concern in drug development, and machine learning models help identify potential adverse effects before clinical trials begin. By analyzing data from previous studies and real-world evidence, AI can predict the toxicity and side effects of new compounds.
- This proactive approach to safety assessment reduces the risk of late-stage trial failures and ensures that only the safest and most effective drugs advance through the development pipeline.
Case Studies: AI in Identifying New Drug Candidates and Optimizing Clinical Trials
- Identifying New Drug Candidates:
- BenevolentAI: This AI-driven biotech company has successfully identified new drug candidates for treating diseases like amyotrophic lateral sclerosis (ALS) and COVID-19. By leveraging machine learning algorithms, BenevolentAI rapidly analyzed vast amounts of biomedical data to uncover novel therapeutic targets and potential drug candidates.
- Insilico Medicine: Using deep learning techniques, Insilico Medicine has identified promising compounds for treating fibrosis and aging-related diseases. Their AI platform accelerates the drug discovery process by predicting the biological activity of new compounds and optimizing their chemical structures for maximum efficacy.
- Optimizing Clinical Trials:
- Atomwise: Atomwise uses AI to optimize clinical trial designs and improve the success rates of new drugs. By predicting how patients will respond to different treatments, Atomwise's machine learning models help select the most suitable candidates for clinical trials, ensuring more efficient and targeted studies.
- GNS Healthcare: GNS Healthcare employs AI to analyze patient data from clinical trials and real-world evidence to identify biomarkers that predict treatment response. Their AI platform helps refine trial protocols, reducing trial duration and increasing the likelihood of regulatory approval.
By harnessing the power of AI, the drug discovery and development process becomes more efficient, cost-effective, and precise. AI-driven approaches not only accelerate the identification of new drug candidates but also enhance the design and execution of clinical trials, ultimately leading to safer and more effective treatments for patients. As AI continues to advance, its integration into drug discovery holds immense potential for transforming the pharmaceutical industry and improving global health outcomes.
Real-Time Monitoring and Adaptive Treatment
AI in Wearable Devices and Remote Monitoring
AI integration into wearable devices and remote monitoring systems has revolutionized real-time health tracking. Wearable devices equipped with sensors collect continuous health data, such as heart rate, blood pressure, and activity levels. AI algorithms then analyze this data to provide insights into the wearer's health status and predict potential health issues.
Machine Learning Models for Continuous Health Tracking
Machine learning models play a crucial role in processing the vast amounts of data generated by wearable devices. These models can identify patterns and anomalies in the data, allowing for early detection of health issues and timely interventions.
- Predictive Analytics: Machine learning algorithms predict health trends and potential problems by analyzing historical and real-time data. For example, in chronic disease management, AI can predict exacerbations and suggest preventive measures.
- Personalized Feedback: AI provides personalized health recommendations based on individual data. For example, it can suggest lifestyle changes, medication adjustments, or the need for medical consultation.
Examples: Real-Time Glucose Monitoring in Diabetes, Remote Patient Management
- Real-Time Glucose Monitoring in Diabetes:
- Continuous Glucose Monitors (CGMs) like the Dexcom G6 and Abbott's FreeStyle Libre use AI to provide real-time glucose readings and trends. These devices help patients with diabetes manage their blood sugar levels more effectively.
- Machine learning models analyze glucose data to predict high and low blood sugar events, allowing patients to take preventive actions. AI-driven insights can also help healthcare providers tailor treatment plans to each patient's needs.
- Remote Patient Management:
- Cardiovascular Health: Wearable devices like smartwatches and fitness trackers monitor heart rate, physical activity, and sleep patterns. AI algorithms analyze this data to detect irregular heart rhythms, such as atrial fibrillation, and alert patients and healthcare providers.
- Chronic Disease Management: Remote monitoring systems for conditions like hypertension, COPD, and heart failure collect data from patients' homes. AI processes this data to detect worsening symptoms and recommend timely interventions, reducing hospital readmissions.
- Telehealth: AI-powered platforms like HealthTap and Babylon Health use machine learning to provide virtual consultations, symptom checking, and personalized health advice. These platforms enhance remote patient management by offering accessible and efficient healthcare services.
AI's role in real-time monitoring and adaptive treatment is transformative. By leveraging wearable devices and advanced machine learning models, AI enables continuous health tracking, early detection of health issues, and personalized care. This approach not only improves patient outcomes but also empowers individuals to take control of their health through timely and actionable insights. As technology advances, the integration of AI into healthcare promises to further revolutionize patient monitoring and management, leading to a more proactive and personalized healthcare system.
Challenges and Ethical Considerations
Data Privacy and Security Concerns
As AI and machine learning become integral to precision medicine, safeguarding patient data becomes paramount. The collection, storage, and analysis of large volumes of sensitive health information introduce significant privacy and security risks.
- Data Breaches: Health data is a prime target for cyberattacks. Ensuring robust cybersecurity measures is crucial to protect against unauthorized access and breaches
- Anonymization and Encryption: Implementing techniques such as data anonymization and encryption can help protect patient identities and secure data during transmission and storage
- Compliance with Regulations: Adhering to regulatory standards such as HIPAA (Health Insurance Portability and Accountability Act) in the US and GDPR (General Data Protection Regulation) in Europe is essential to ensure data privacy and security
Ensuring Fairness and Mitigating Biases in AI Algorithms
AI algorithms can inadvertently perpetuate biases present in the training data, leading to unfair or inaccurate outcomes. Addressing these biases is critical for the equitable application of AI in healthcare.
- Diverse and Representative Data: Ensuring that training datasets are diverse and representative of different populations can help mitigate biases. This includes considering factors such as age, gender, ethnicity, and socioeconomic status.
- Bias Detection and Correction: Developing methods for detecting and correcting biases in AI models is essential. Regular audits and updates to algorithms can help maintain fairness and accuracy.
- Transparency and Explainability: AI systems should be transparent and their decision-making processes explainable. This helps build trust and allows for the identification and correction of potential biases.
Regulatory and Ethical Frameworks for AI in Healthcare
The integration of AI in healthcare necessitates robust regulatory and ethical frameworks to ensure safe, effective, and ethical use of these technologies.
- Regulatory Approvals: Establishing clear guidelines for the development, testing, and approval of AI-based healthcare solutions is crucial. Regulatory bodies such as the FDA (Food and Drug Administration) in the US play a key role in this process.
- Ethical Guidelines: Developing ethical guidelines for the use of AI in healthcare can address issues such as informed consent, patient autonomy, and the ethical implications of AI-driven decisions.
- Interdisciplinary Collaboration: Collaboration between AI developers, healthcare professionals, ethicists, and regulators is essential to create comprehensive and effective frameworks. This ensures that AI technologies are developed and deployed in a manner that prioritizes patient welfare and adheres to ethical standards.
In conclusion, while AI and machine learning hold great promise for enhancing precision medicine, addressing challenges related to data privacy, fairness, and ethical use is essential. By implementing robust security measures, ensuring diverse and unbiased data, and adhering to regulatory and ethical guidelines, the healthcare industry can harness the full potential of AI to improve patient outcomes and advance medical research responsibly.
Future Directions and Innovations
Emerging Trends in AI and Machine Learning for Precision Medicine
As technology continues to advance, several emerging trends are set to further enhance the capabilities of AI and machine learning in precision medicine:
- Integration of Multi-Omics Data: Combining genomics, proteomics, metabolomics, and other omics data allows for a more comprehensive understanding of disease mechanisms and patient-specific factors, leading to highly personalized treatment strategies
- Federated Learning: This approach enables machine learning models to be trained across multiple decentralized data sources without transferring data, thus enhancing data privacy and security while leveraging diverse datasets
- Natural Language Processing (NLP): NLP techniques are being increasingly used to analyze unstructured clinical data from electronic health records (EHRs), medical literature, and patient feedback, providing deeper insights and aiding in clinical decision-making
- Explainable AI (XAI): Developing AI systems that can provide transparent and understandable explanations for their decisions helps build trust among clinicians and patients, ensuring the practical and ethical application of AI in healthcare
- AI-Driven Predictive Analytics: Predictive analytics powered by AI can forecast disease outbreaks, patient deterioration, and treatment outcomes, allowing for proactive and preventive healthcare measures
Potential Breakthroughs and Their Impact on Patient Care
The continued advancement of AI and machine learning technologies promises several breakthroughs that could revolutionize patient care:
- Real-Time Personalized Treatment: AI systems capable of processing real-time data from wearable devices and other monitoring tools can provide instant insights and adjustments to treatment plans, ensuring optimal patient outcomes
- Precision Oncology: AI can identify specific genetic mutations and biomarkers in cancer patients, enabling the development of highly targeted therapies that significantly improve treatment efficacy and reduce side effects
- Remote and Telehealth Services: AI-enhanced telehealth platforms can offer remote diagnosis, treatment recommendations, and continuous patient monitoring, making healthcare more accessible and efficient, especially in underserved areas
- Chronic Disease Management: AI algorithms can monitor and predict the progression of chronic diseases, helping patients and healthcare providers manage conditions more effectively and prevent complications
Collaborative Efforts Between AI Researchers, Clinicians, and Healthcare Providers
The successful integration of AI and machine learning into precision medicine requires collaboration across various stakeholders:
- Interdisciplinary Collaboration: Close collaboration between AI researchers, clinicians, and healthcare providers ensures that AI solutions are clinically relevant, practical, and aligned with patient needs
- Public-Private Partnerships: Partnerships between academic institutions, healthcare organizations, and technology companies can drive innovation, provide funding, and facilitate the translation of research into real-world applications
- Education and Training: Investing in the education and training of healthcare professionals in AI technologies is crucial for the effective adoption and utilization of AI tools in clinical practice
- Patient Involvement: Engaging patients in the development and implementation of AI-driven healthcare solutions ensures that these technologies address patient concerns and improve the overall patient experience
- Regulatory and Ethical Oversight: Ongoing dialogue between regulators, ethicists, and technology developers is necessary to create robust frameworks that safeguard patient interests and promote the ethical use of AI in healthcare.
In conclusion, the future of precision medicine is poised to be significantly enhanced by the integration of AI and machine learning. By leveraging emerging trends, fostering interdisciplinary collaboration, and ensuring ethical and equitable implementation, the healthcare industry can harness the full potential of these technologies to improve patient care, drive medical innovation, and achieve better health outcomes for all.
Recap of the Transformative Role of AI and Machine Learning in Precision Medicine
AI and machine learning have proven to be game-changers in the field of precision medicine. These technologies have revolutionized the way we approach data analysis, diagnostic accuracy, personalized treatment plans, drug discovery, real-time monitoring, and adaptive treatment. By processing vast amounts of data and identifying patterns that were previously undetectable, AI has opened up new avenues for more accurate, efficient, and personalized healthcare.
As we stand on the brink of a new era in medicine, it is essential to continue pushing the boundaries of research and innovation. The potential for AI and machine learning to transform healthcare is immense, but it requires sustained investment, collaboration, and creativity. Researchers, clinicians, and tech developers must work together to refine these technologies and expand their applications, ensuring they deliver the maximum possible benefit to patients.
The future of precision medicine is bright, with AI and machine learning at its core. These technologies promise to make healthcare more proactive, predictive, and personalized, significantly improving patient outcomes. However, realizing this potential requires a concerted effort to address current challenges, including data privacy, ethical considerations, and the need for robust regulatory frameworks.
Staying informed about the latest advancements in AI and precision medicine is crucial for all stakeholders, from healthcare professionals to patients and policymakers. By keeping up-to-date with new research, technologies, and best practices, we can ensure that the integration of AI into healthcare continues to evolve and improve.
Investment in AI research and its application in healthcare is vital. Stakeholders, including governments, private enterprises, and philanthropic organizations, are encouraged to support initiatives that drive innovation and development in this field. By doing so, we can accelerate the pace of discovery and implementation, bringing the benefits of AI-driven precision medicine to patients more quickly.
As we integrate AI more deeply into healthcare, it is essential to maintain a strong focus on ethical and responsible practices. Ensuring patient privacy, addressing biases in AI algorithms, and promoting transparency are all critical components of building trust and achieving equitable outcomes. Ethical oversight and robust regulatory frameworks will play a pivotal role in guiding the responsible use of AI in medicine.
In conclusion, AI and machine learning are set to redefine precision medicine, offering unprecedented opportunities to improve patient care and outcomes. Through continued research, collaboration, and ethical practices, we can harness the full potential of these technologies, ushering in a new era of healthcare that is more personalized, efficient, and effective.
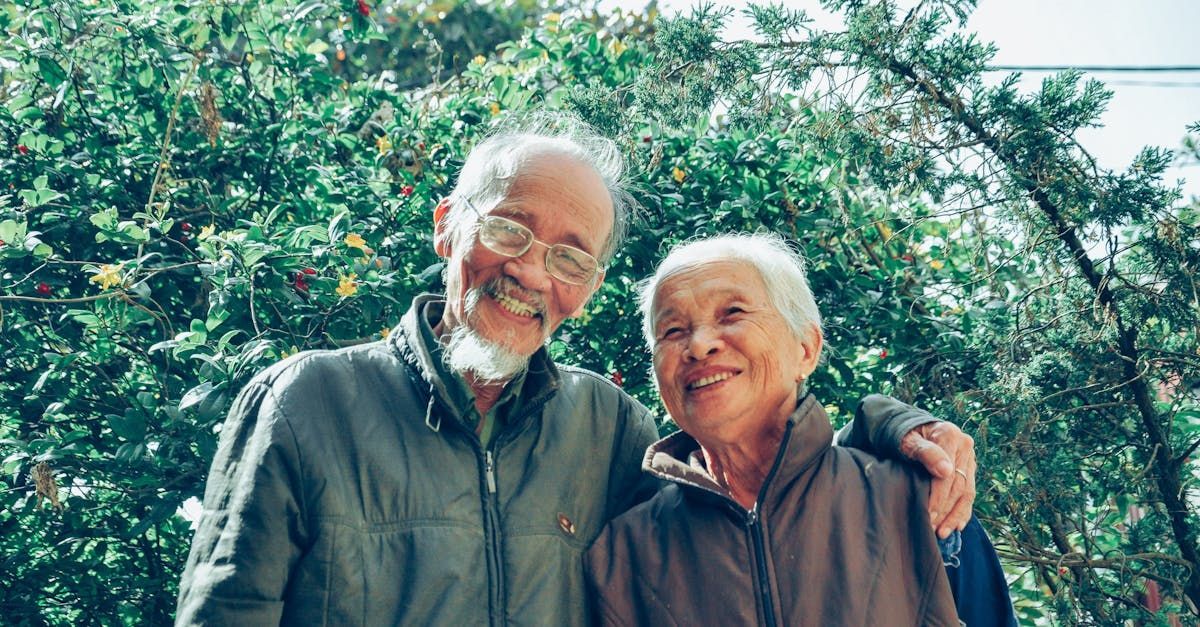
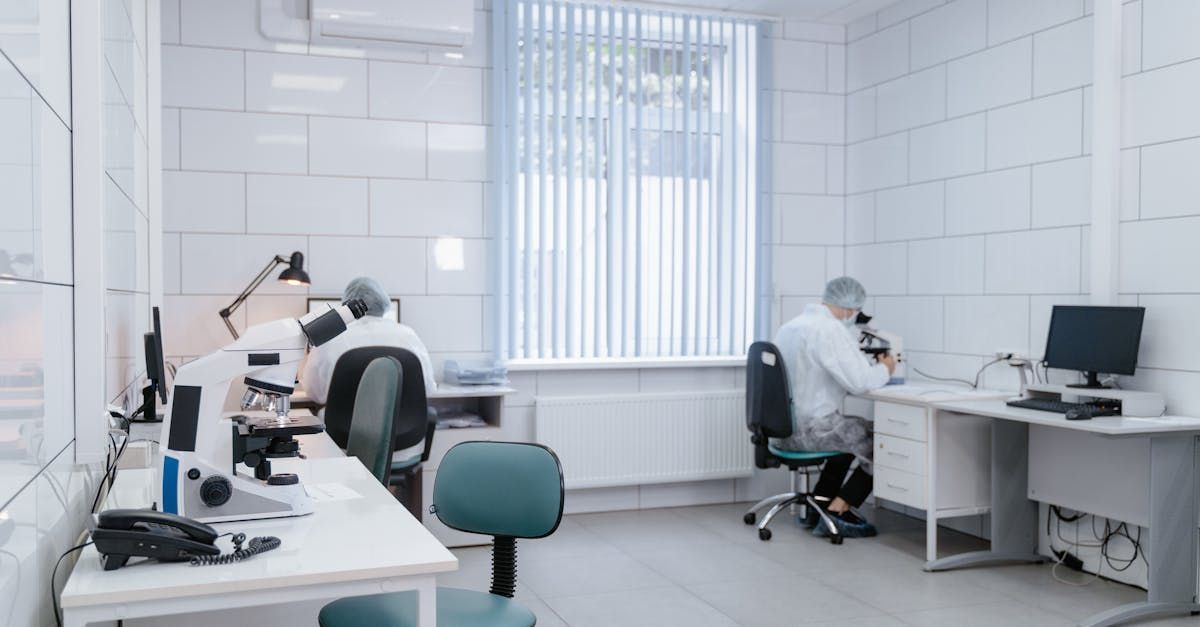